Research
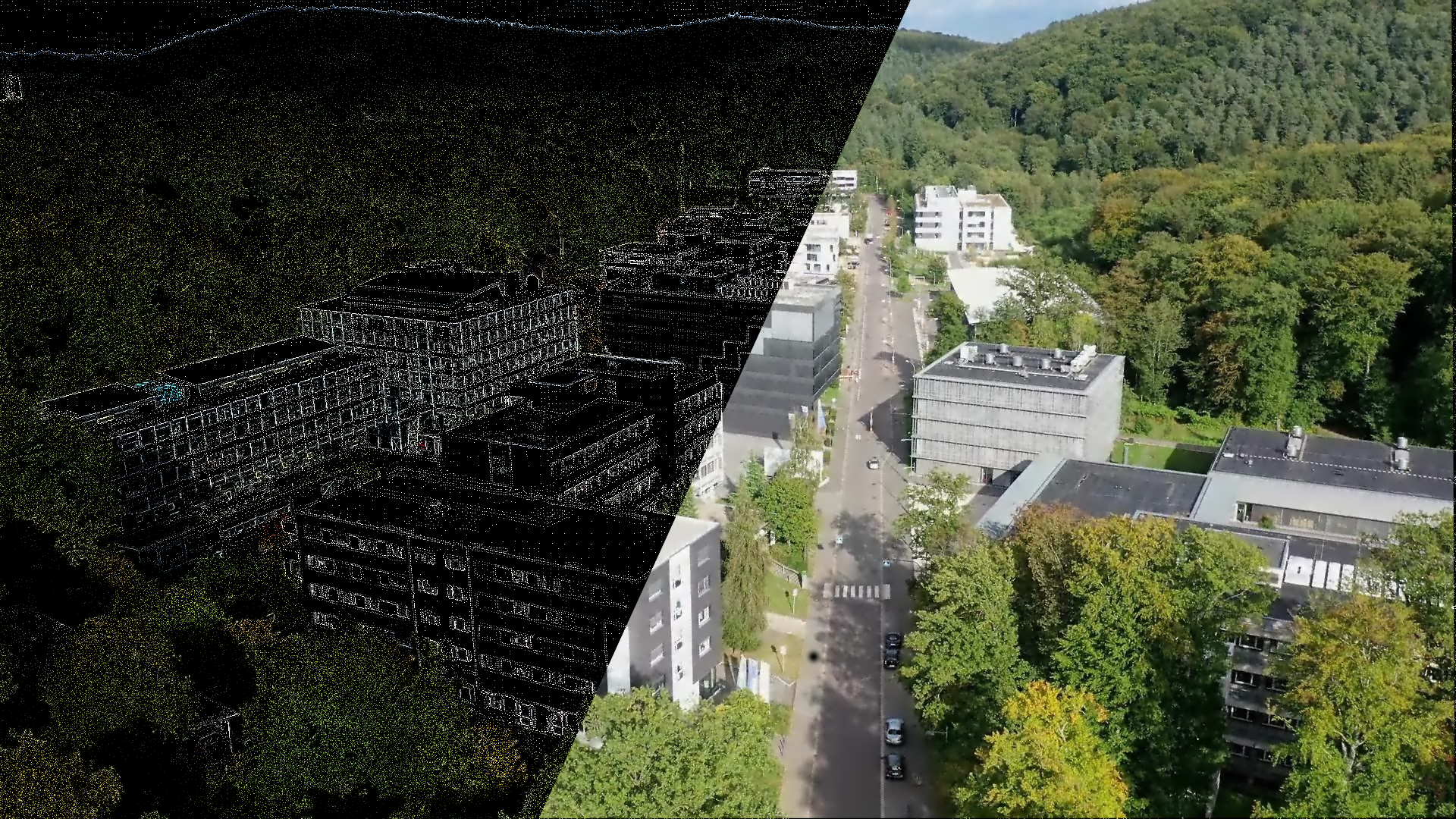
Research Interests
- Image Inpainting: Reconstruction of missing image data.
- Sparse Data Optimisation: Selecting image pixels or other sparse representations for high quality reconstructions.
- Image Compression: Storing images at high fidelity at low coding cost.
- Mathematical Foundations of Deep Learning: Finding mathematical interpretations for existing deep learning approaches or inspiring deep learning models from mathematics.
- Scale-Space Theory: Embedding images into hiearchical families of gradual simplifications.
Publications
D. Gaa, V. Chizhov, P. Peter, J. Weickert, R. D. Adam:
Connecting image inpainting with denoising in the homogeneous diffusion setting.
Advances in Continuous and Discrete Models, Vol. 2025, Article No. 74, 2025P. Peter:
Generalised diffusion probabilistic scale-spaces.
Journal of Mathematical Imaging and Vision, Vol. 66, 639-656, June 2024.
Invited Paper.P. Peter, K. Schrader, T. Alt, J. Weickert:
Deep spatial and tonal optimisation for homogeneous diffusion inpainting.
Pattern Analysis and Applications, Vol. 26, No. 4, 1585-1600, November 2023.
Invited Paper.T. Alt, K. Schrader, M. Augustin, P. Peter, J. Weickert:
Connections between numerical algorithms for PDEs and neural networks.
Journal of Mathematical Imaging and Vision, June 2022.
Invited Paper.
Also available as arXiv:2107.14742 [math.NA], revised March 2022.T. Alt, K. Schrader, J. Weickert, P. Peter, M. Augustin:
Designing rotationally invariant neural networks from PDEs and variational methods.
Research in the Mathematical Sciences, Vol. 9, No. 3, Article 52, Sept. 2022.
Also available as arXiv:2108.13993 [cs.LG], revised March 2022.R. M. K. Mohideen, P. Peter, J. Weickert:
A systematic evaluation of coding strategies for sparse binary images.
Signal Processing: Image Communication, Vol. 99, Article 116424, November 2021.
Also available as arXiv:2010.13634 [eess.IV], revised July 2021.M. Breuß, J. Buhl, A. M. Yarahmadi, M. Bambach, P. Peter:
A simple approach to stiffness enhancement of a printable shape by Hamilton-Jacobi skeletonization.
Procedia Manufacturing, Vol. 47, 1190-1196, 2020.- L. Hoeltgen, P. Peter, M. Breuß:
Clustering-Based Quantisation for PDE-Based Image Compression.
Signal, Image and Video Processing, Vol. 12, No. 3, 411-419, Vol. 12, No. 3, 411-419 March 2018.
Revised version of arXiv:1706.06347 [cs.CV], June 2017 N. Amrani, J. Serra-Sagrista, P. Peter, J. Weickert:
Diffusion-based inpainting for coding remote-sensing data.
IEEE Geoscience and Remote Sensing Letters, Vol. 14, No. 8, 1203-1207, August 2017.
Also available as Technical Report, Universitat Autonoma de Barcelona, Spain, March 2017, ddd.uab.cat/record/174184.P. Peter, L. Kaufhold, J. Weickert:
Turning diffusion-based image colorization into efficient color compression.
IEEE Transactions on Image Processing, Vol. 26, No. 2, 860-869, February 2017.
Revised version of Technical Report No. 370, Department of Mathematics, Saarland University, Saarbrücken, Germany, December 2015.P. Peter, S. Hoffmann, F. Nedwed, L. Hoeltgen, J. Weickert:
Evaluating the true potential of diffusion-based inpainting in a compression context.
Signal Processing: Image Communication, Vol. 46, 40-53, August 2016.
Revised version of Technical Report No. 373, Department of Mathematics, Saarland University, Saarbrücken, Germany, January 2016.P. Peter, C. Schmaltz, N. Mach, M. Mainberger, J. Weickert:
Beyond Pure Quality: Progressive Modes, Region of Interest Coding, and Real Time Video Decoding for PDE-based Image Compression.
Journal of Visual Communication and Image Representation, Vol. 31, 253-265, August 2015.
Revised version of Technical Report No. 354, Department of Mathematics, Saarland University, Saarbrücken, Germany, January 2015.C. Schmaltz, P. Peter, M. Mainberger, F. Ebel, J. Weickert, A. Bruhn:
Understanding, optimising, and extending data compression with anisotropic diffusion.
International Journal of Computer Vision, Vol. 108, No. 3, 222-240, July 2014.
Revised version of Technical Report No. 329, Department of Mathematics, Saarland University, Saarbrücken, Germany, March 2013.
- P. Peter and M. Breuß
Refined Homotopic Thinning Algorithms and Quality Measures for Skeletonisation Methods.
M. Breuß, A. Bruckstein, P. Maragos (Eds.): Innovations for Shape Analysis: Models and Algorithms. Mathematics and Visualization, 77-92, Springer, Berlin, 2013.
Revised version of Technical Report No. 312, Department of Mathematics, Saarland University, Saarbrücken, Germany, July 2012.
J. Gierke, P. Peter:
Skeletonisation scale-spaces.
To appear in Scale Space and Variational Methods in Computer Vision. Lecture Notes in Computer Science, Springer, Cham, 2025.
Also available as arXiv:2503.03450 [eess.IV], March 2025.T. Fischer, P. Peter, J. Weickert, E. Ilg:
Neuroexplicit diffusion models for inpainting of optical flow fields.
Proc. 41st International Conference on Machine Learning (ICML 2024, Vienna, Austria, July 2024), Proceedings of Machine Learning Research, Vol. 235, 13691-13705, 2024P. Bungert, P. Peter, J. Weickert:
Image blending with osmosis.
In L. Calatroni, M. Donatelli, S. Morigi, M. Prato, M. Santavesaria (Eds.): Scale Space and Variational Methods in Computer Vision. Lecture Notes in Computer Science, Vol. 14009, Springer, Cham, 652-664, 2023.
Also available as arXiv:2303.07762 [eess.IV], March 2023.P. Peter:
Generalised scale-space properties for probabilistic diffusion.
In L. Calatroni, M. Donatelli, S. Morigi, M. Prato, M. Santavesaria (Eds.): Scale Space and Variational Methods in Computer Vision. Lecture Notes in Computer Science, Vol. 14009, Springer, Cham, 601-613, 2023.
Also available as arXiv:2303.07900 [eess.IV], March 2023.K. Schrader, P. Peter, N. Kämper, J. Weickert:
Efficient neural generation of 4K masks for homogeneous diffusion inpainting.
In L. Calatroni, M. Donatelli, S. Morigi, M. Prato, M. Santavesaria (Eds.): Scale Space and Variational Methods in Computer Vision. Lecture Notes in Computer Science, Vol. 14009, Springer, Cham, 16-28, 2023.
Also available as arXiv:2303.10096 [eess.IV], March 2023.P. Peter:
A Wasserstein GAN for joint learning of inpainting and its spatial optimisation.
In H. Wang, W. Lin, P. Manoranjan, G. Xiao, K.L. Chan, X. Wang, G. Ping, H. Jiang: Image and Video Technology. Lecture Notes in Computer Science, Vol. 13763, Springer, Cham, 132-145, 2023.
Also available as arXiv:2202.05623 [eess.IV], revised December 2022.T. Alt, P. Peter, J. Weickert:
Learning sparse masks for diffusion-based image inpainting.
In A. J. Pinho, P. Georgieva, L. F. Teixeira, J. A. Sánchez (Eds.): Pattern Recognition and Image Analysis. Lecture Notes in Computer Science, Vol. 13256, Springer, Cham, 528-539, 2022.
Also available as arXiv:2110.02636 [eess.IV], revised March 2022.S. Andris, J. Weickert, T. Alt, P. Peter:
JPEG meets PDE-based image compression.
In Proc. 35th Picture Coding Symposium (PCS 2021, Bristol, UK, June 2021), IEEE Press, 2021.
Also available as arXiv:2011.11289 [eess.IV], revised May 2021.P. Peter:
Quantisation scale-spaces.
In A. Elmoataz, J. Fadili, Y. Quéau, J. Rabin, L. Simon (Eds.): Scale Space and Variational Methods in Computer Vision. Lecture Notes in Computer Science, Vol. 12679, Springer, Cham, 15-26, 2021.
Also available as arXiv:2103.10491 [eess.IV], March 2021.T. Alt, P. Peter, J. Weickert, K. Schrader:
Translating numerical concepts for PDEs into neural architectures.
In A. Elmoataz, J. Fadili, Y. Quéau, J. Rabin, L. Simon (Eds.): Scale Space and Variational Methods in Computer Vision. Lecture Notes in Computer Science, Vol. 12679, Springer, Cham, 294-306, 2021.
Also available as arXiv:2103.15419 [math.NA], March 2021.S. Andris, P. Peter, R. M. K. Mohideen, J. Weickert, S. Hoffmann:
Inpainting-based video compression in FullHD.
In A. Elmoataz, J. Fadili, Y. Quéau, J. Rabin, L. Simon (Eds.): Scale Space and Variational Methods in Computer Vision. Lecture Notes in Computer Science, Vol. 12679, Springer, Cham, 425-436, 2021.
Also available as arXiv:2008.10273 [eess.IV], revised May 2021.F. Jost, P. Peter, J. Weickert:
Compressing piecewise smooth images with the Mumford-Shah cartoon model.
In Proc. 28th European Signal Processing Conference (EUSIPCO 2020, Amsterdam, Netherlands, January 2021), 511-515, 2021.
Also available as arXiv:2003.05206 [eess.IV], March 2020.F. Jost, P. Peter, J. Weickert:
Compressing flow fields with edge-aware homogeneous diffusion inpainting.
Proc. 45th International Conference on Acoustics, Speech, and Signal Processing (ICASSP 2020, Barcelona, Spain, May 2020), 2198-2202, 2020.
Also available as arXiv:1906.12263 [eess.IV], October 2019.P. Peter:
Fast inpainting-based compression: Combining Shepard interpolation with joint inpainting and prediction.
Proc. 2019 IEEE International Conference on Image Processing (ICIP 2019, Taipei, Taiwan, Sept. 2019), 3557-3561, 2019.M. Cárdenas, P. Peter, J. Weickert:
Sparsification scale-spaces.
In J. Lellmann, M. Burger, J. Modersitzki (Eds.): Scale Space and Variational Methods. Lecture Notes in Computer Science, Vol. 11603, 303-314, Springer, Cham, 2019.P. Peter, J. Contelly, J. Weickert:
Compressing audio signals with inpainting-based sparsification.
In J. Lellmann, M. Burger, J. Modersitzki (Eds.): Scale Space and Variational Methods. Lecture Notes in Computer Science, Vol. 11603, 92-103, Springer, Cham, 2019.L. Karos, P. Bheed, P. Peter, J. Weickert:
Optimising data for exemplar-based inpainting.
In J. Blanc-Talon, D. Helbert, W. Philips, D. Popescu, P. Scheunders (Eds.): Advanced Concepts for Intelligent Vision Systems. Lecture Notes in Computer Science, Vol. 11182, 547-558, Springer, Cham, 2018.- R. D. Adam, P. Peter, J. Weickert:
Denoising by inpainting.
In F. Lauze, Y. Dong, A. B. Dahl (Eds.): Scale Space and Variational Methods in Computer Vision. Lecture Notes in Computer Science, Vol. 10302, 121-132, Springer, Cham, 2017.
- S. Andris, P. Peter, J. Weickert:
A proof-of-concept framework for PDE-based video compression.
Proc. 32nd Picture Coding Symposium (PCS 2016, Nuremberg, Germany, December. 2016), 1-5, 2016.
PCS 2016 Best Poster Award.
- M. Schneider, P. Peter, S. Hoffmann, J. Weickert, Enric Meinhardt-Llopis:
Gradients versus grey values for sparse image reconstruction and inpainting-based compression.
In J. Blanc-Talon, C. Distante, W. Philips, D. Popescu, P. Scheunders (Eds.): Advanced Concepts for Intelligent Vision Systems. Lecture Notes in Computer Science, Vol. 10016, 1-13, Springer, Cham, 2016.
- P. Peter, S. Hoffmann, F. Nedwed, L. Hoeltgen, J. Weickert:
From optimised inpainting with linear PDEs towards competitive image compression codecs.
In T. Bräunl, B. McCane, M. Rivera, X. Yu (Eds.): Image and Video Technology. Lecture Notes in Computer Science, Vol. 9431, 63-74, Springer, Cham, 2016.
- P. Peter, J. Weickert:
Compressing images with diffusion- and exemplar-based inpainting.
In J.-F. Aujol, M. Nikolova, N. Papadakis (Eds.): Scale Space and Variational Methods in Computer Vision. Lecture Notes in Computer Science, Vol. 9087, 154-165, Springer, Berlin, 2015.
- P. Peter, J. Weickert, A. Munk, T. Krivobokova, H. Li:
Justifying tensor-driven diffusion from structure-adaptive statistics of natural images.
In X.-C. Tai, E. Bae, T. F. Chan, M. Lysaker (Eds.): Energy Minimization Methods in Computer Vision and Pattern Recognition. Lecture Notes in Computer Science, Springer, Vol. 8932, 263-277, Berlin, 2015.
- P. Peter, J. Weickert:
Colour image compression with anisotropic diffusion.
Proc. 21st IEEE International Conference on Image Processing
(ICIP 2014, Paris, France, October 2014), 4822-4826, 2014.
- P. Peter
Three-dimensional data compression with anisotropic diffusion.
J. Weickert, M. Hein, B. Schiele (Eds.): Pattern Recognition. Lecture Notes in Computer Science, Volume 8142, 231-236, Springer, Berlin, 2013.
Verantwortlich für die Inhalte dieses Webangebots
Dr. Pascal Peter
Akademischer Rat
Campus E2 4, 66123 Saarbrücken
Tel.: 0681 302-58096
peter(at)math.uni-saarland.de