Special issue on novel applications of learning curves in production planning and logistics
Kurzbeschreibung
This special issue in Computers & Industrial Engineering aims to publish innovative approaches for the integration of learning and forgetting curves into mathematical models, simulation approaches or decision support models with a special focus on logistics and production and operations management. The special issue looks especially for works that develop quantitative models based on empirically collected data on learning and forgetting. In addition, authors are invited to submit novel applications of learning curves in particular for specific industries or environments (individual, group and organizational learning).
Publikationen
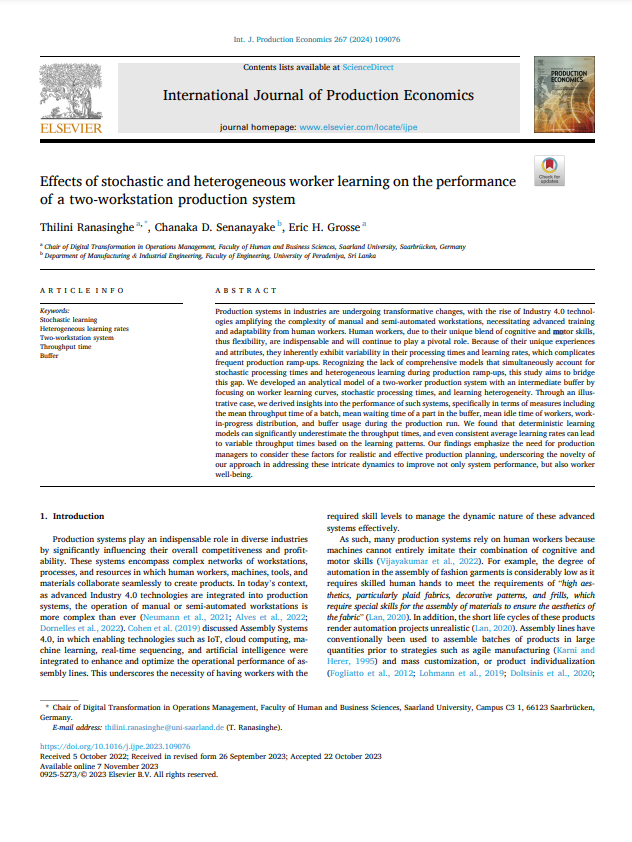
Effects of stochastic and heterogeneous worker learning on the performance of a two-workstation production system
Ranasinghe, Senanayake & Grosse (2024)
Production systems in industries are undergoing transformative changes, with the rise of Industry 4.0 technologies amplifying the complexity of manual and semi-automated workstations, necessitating advanced training and adaptability from human workers. Human workers, due to their unique blend of cognitive and motor skills, thus flexibility, are indispensable and will continue to play a pivotal role. Because of their unique experiences and attributes, they inherently exhibit variability in their processing times and learning rates, which complicates frequent production ramp-ups. Recognizing the lack of comprehensive models that simultaneously account for stochastic processing times and heterogeneous learning during production ramp-ups, this study aims to bridge this gap. We developed an analytical model of a two-worker production system with an intermediate buffer by focusing on worker learning curves, stochastic processing times, and learning heterogeneity. Through an illustrative case, we derived insights into the performance of such systems, specifically in terms of measures including the mean throughput time of a batch, mean waiting time of a part in the buffer, mean idle time of workers, workin-progress distribution, and buffer usage during the production run. We found that deterministic learning models can significantly underestimate the throughput times, and even consistent average learning rates can lead to variable throughput times based on the learning patterns. Our findings emphasize the need for production managers to consider these factors for realistic and effective production planning, underscoring the novelty of our approach in addressing these intricate dynamics to improve not only system performance, but also worker well-being.
Production economics and the learning curve: A meta-analysis
Grosse, Glock & Müller (2015)
International Journal of Production Economics 170
For almost a century, researchers and practitioners have studied learning curves in production economics. Learning, in this context, refers to performance improvements of individuals, groups or organizations over time as a result of accumulated experience. Various learning curves, which model this phenomenon, have been developed and applied in the area of production economics in the past. When developing planning models in production economics, the question arises which learning curve should be used to best describe the learning process. In the past, the focus of the literature has been on empirical studies that investigated learning processes in laboratory settings or in practice, but no effort has been undertaken so far to compare existing learning curves on a large set of empirical data to assess which learning curve should be used for which application. This study systematically collected empirical data on learning curves, which resulted in a large database of empirical data on learning. First, the data contained in the database is categorized with the help of meta-tags along different characteristics of the studies the data was taken from. Second, a selection of well-known learning curves is fitted to the empirical datasets and analyzed with regard to goodness of fit and data characteristics. We identify a set of data/task characteristics that are important for selecting an appropriate learning curve. The results of the paper may be used in production economics to assist researchers to select the right learning curve for their modeling efforts.
Applications of learning curves in production and operations management: A systematic literature review
Glock, Grosse, Jaber & Smunt (2018)
Computers & Industrial Engineering
The occurrence and characteristics of human learning and forgetting are extensively researched in many fields. Thus, the literature on learning curves is abundant. Within production and operations management, learning curves can describe the performance improvement of workers due to repetitions or experience, which makes them a useful tool for managerial decision making. Human learning is very relevant to manufacturing firms that are labor-intensive, especially where labor is costly. Also, technology and organization learning frequently occur in many organizations, even when manufacturing is not labor-intensive. It is not surprising, therefore, that, despite the plethora of prior work in learning curves, research in this important area continues to appear in the production and operations management literature. A comprehensive, systematic review of the literature, however, has never been conducted. This article aims at giving a general overview of learning curves in production and operations management. It maps this specific research area, synthesizes its research findings, highlights its key application areas and explores future research directions. This work achieves this goal by presenting the results of a systematic literature review on the applications of learning curves in production and operations management. First, a framework that includes typical learning curve models is developed. The fundamental characteristics of learning curves and their applications in production and operations management are then identified. This framework is used to categorize the literature. This study also presents a discussion of the most important and informative articles in each of the major categories, as well as highlighting future research opportunities.
Editorial: Novel applications of learning curves in production planning and logistics
Glock, Grosse, Jaber & Smunt (2019)
Computers & Industrial Engineering
Workforce demographics and work contents are changing. In most developed economies, the average age of the workforce is also increasing. The cost of retaining skilled labour is becoming expensive, tasks more complex, and alternating between tasks is getting more common. In such environments, the development, management, and acquisition and retention of knowledge are essential for driving and sustaining the competitive position of a firm in a market (e.g., Beechler & Woodward, 2009; Myerson, Bichard, & Erlich, 2010).
Adjustment for cognitive interference enhances the predictability of the power learning curve
Jaber, Peltokorpi, Glock, Grosse & Pusic (2021)
International Journal of Production Economics
Learning curves, which express performance as a function of the cumulative number of repetitions when performing a given task, have a long tradition of supporting managerial decisions in production and operations management. Performance generally improves as the number of repetitions of a given task increases, with the latter being a primary proxy to reflect experience. A learning curve is usually a maximum-likelihood trend-line that best fits raw data points by splitting them to above and below it. However, its curvature does not always accurately capture the scatter around it, which reduces its accuracy. This paper advocates for an improved learning curve, one that accounts for the variable degree of cognitive interference that occurs while learning when moving from one repetition to the next. To capture this phenomenon, this paper accounts for memory traces of repetitions to measure the residual (interference-adjusted), not the nominal (maximum), cumulative experience. Two alternative learning curve models were developed. The first model aggregates the residual cumulative experience for each repetition while fitting the data. The second model is an approximate expression and, as a continuous model, much easier to implement. The models were tested against data from different learning environments (such as production and assembly), alongside a more traditional power (log-linear) form of the learning curve and its plateau version. The results show that the interference-adjusted models fit the data very well, such that they can serve as valuable tools in production and operations management.